Text: James Woodcock, 4 November 2024
An overview of the current state of artificial intelligence in Additive Manufacturing with a special focus on the areas of idea generation and design, the construction process, quality assurance and future error prevention.
AI, as you may have noticed, is everywhere. Whether it is a discussion about political elections, the implications for recruitment, education or for manufacturing, AI is seemingly always a key part of the conversation. Applications of AI models are nascent across all use cases, including within AM. In this area, the National Institute of Standards and Technology (NIST) is currently running the Advanced Informatics and Artificial Intelligence for Additive Manufacturing (AI2AM) project to develop the methods, models, standards, and best practices to leverage AI and related technologies to move AM towards “born qualified” and “first part correct” outcomes.
Despite the fledgling nature of much of the technology, in the world of AM, different types of AI do, will or could find use across the process chain.
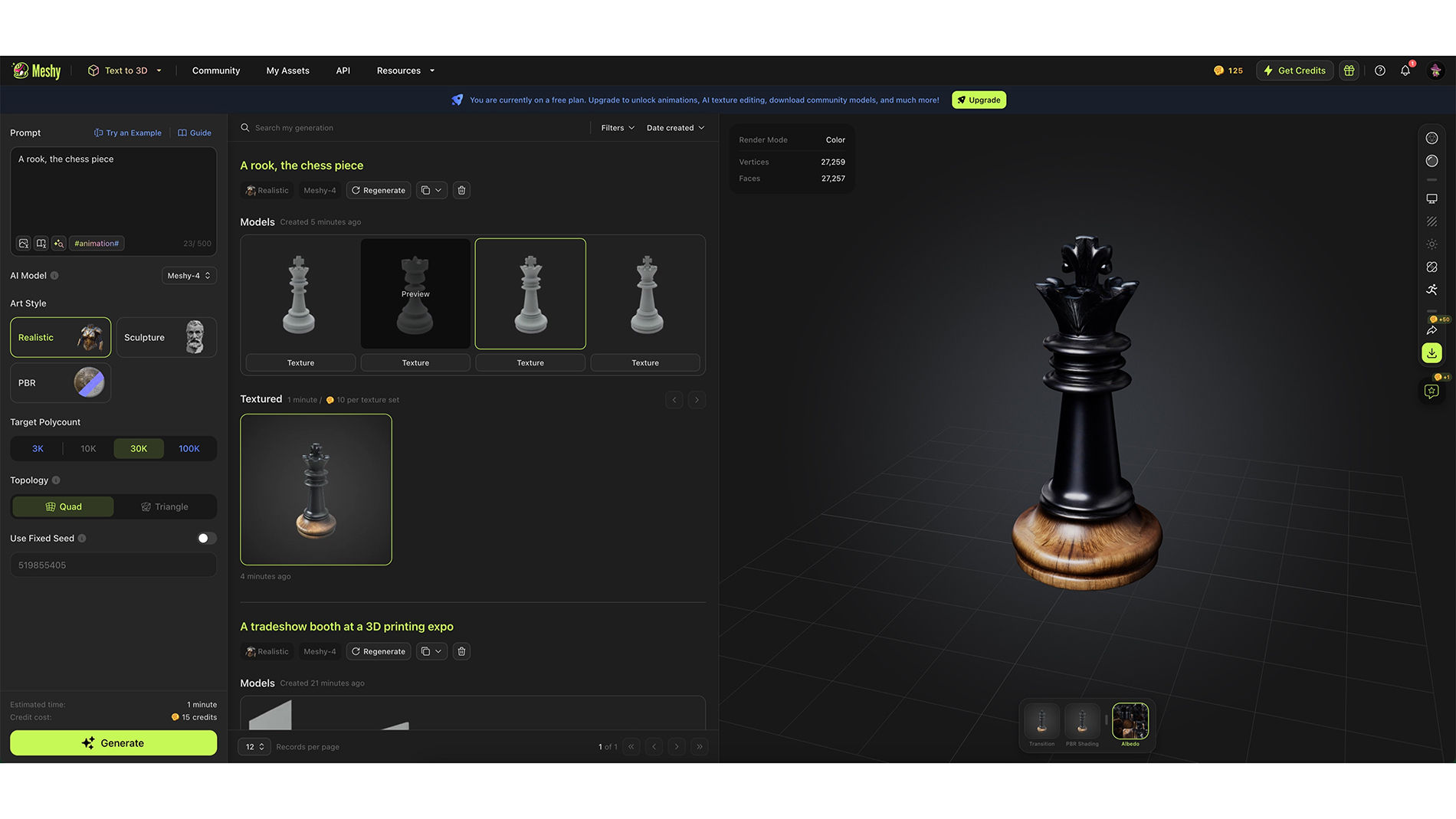
Ideation and design
AI can play a significant role in the DfAM space, with generative AI able to create complex designs that make full use of the benefits of AM. As well as being embedded in major CAD software like Autodesk Fusion 360 and Siemens NX, standalone products like Meshy AI allows users to create 3D models — exportable as .stl files — from either text prompts or photographs. Deploying deep learning and generative design in this way drastically reduces the design time while allowing more solutions to be trialed in a reduced timeframe.
Once part files have been generated, several AI processes including machine learning, predictive analytics and physics-informed AI software can then be used to analyze and fix files prior to printing. By analyzing the parts and mining known datasets about how different AM systems build, solutions like 1000 Kelvin’s AMAIZE, can predict issues with individual builds based on the part geometry, machine parameters and materials choice, reducing the number of failed builds.
Here is a concrete example: The Citrine Platform was used in collaboration with the Alliance for the Development of Additive Processing Technologies (ADAPT) Consortium to optimize the design and production of hinges for Mine-Resistant Ambush Protected (MRAP) light tactical vehicles. AI-driven optimization led to a 50% increase in strength, 38% reduction in weight, and consolidated the original six parts down to one.
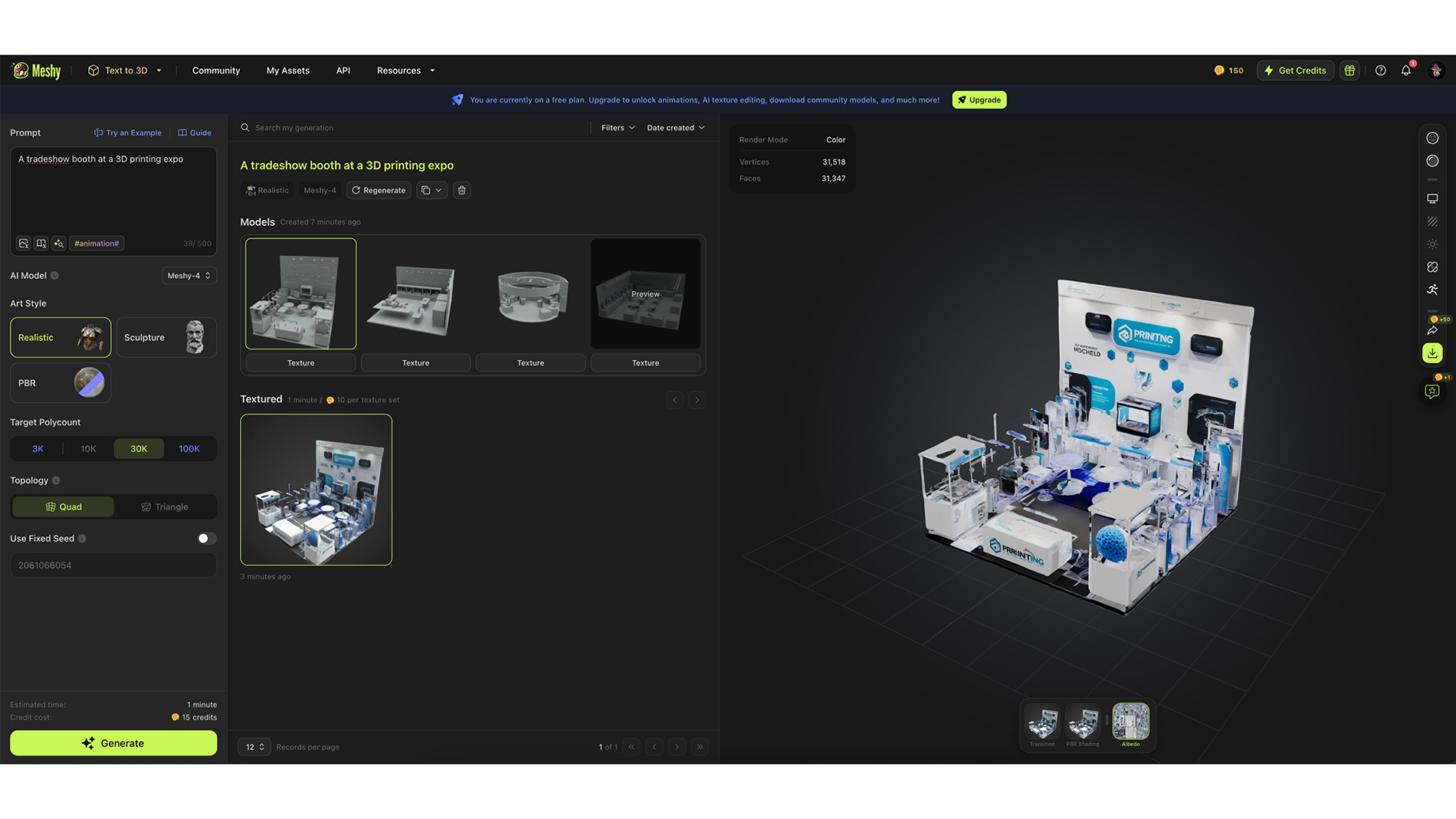
Defining the build
Machine learning (ML) models can analyze huge material datasets and combine that information with data from previous builds to predict how a given material will behave during the printing process. This allows engineers to make informed decisions about which materials to use for an application based on real-world data.
Predictive analytics AI ensures that schedules are optimized for maximum efficiency, helping manufacturers reduce downtime by planning and scheduling optimal times to run certain print jobs, based on machine availability, material stocks, and job priorities.
AI as QA
AM processes, especially in metals, require the complex choreography of chemistry and physics. Things can and do go wrong. AI-based computer vision systems use deep learning to track each layer of a print as it is being produced, flagging defects such as layer shifts, warping, or other inconsistencies in real-time. Where fixes can be implemented, feedback loops can action corrections, at the very least part builds can be stopped at the point of failure instead of letting the process run.
Once the parts are built, inspection is still required — a time-consuming process open to human error. AI-driven automated inspection systems solve this by using computer vision and deep learning to analyze parts post-production. Companies like EOS have integrated computer vision into their platforms to provide real-time process monitoring (EOSCONNECT), ensuring that defects are identified early in the process. Oqton’s Build Quality combines build simulation, monitoring, and part inspection to ensure parts are built to specification without human interference.
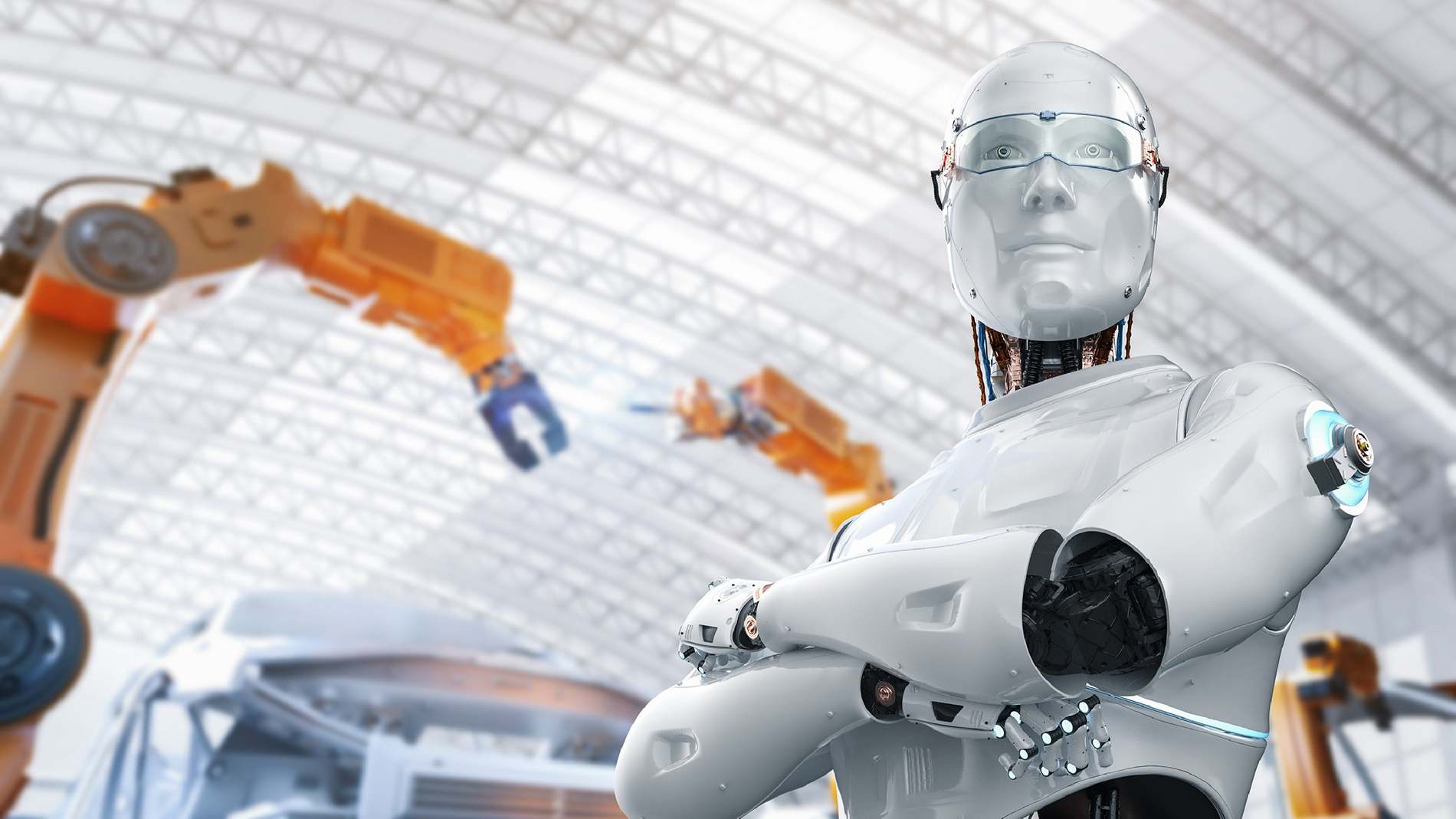
Perennial bugbear, post-processing, can also be automated with AI-driven robotic systems, further optimizing the production workflow, and ensuring consistent results by handling support removal and surface finishing.
Future failure alert
Predictive maintenance — i.e., fixing a system before it breaks — is one of the most significant areas where AI adds value to users of AM systems. By using machine learning and IoT/IIoT-enabled sensors, AI can monitor the health of AM machines and predict when maintenance is required before a breakdown occurs. This proactive approach minimizes unplanned downtime, reduces repair costs, and extends machine lifespan. By integrating predictive maintenance solutions, manufacturers can achieve 20% efficiency gains and increase machine utilization, significantly enhancing profitability.
Explanation of terms
Machine Learning
A subset of AI focused on building systems that learn from data to make predictions or decisions without being explicitly programmed for each task.
Deep Learning
A subset of machine learning that uses neural networks to model complex patterns in data. Deep learning is particularly effective for tasks like image and speech recognition.
Generative Models
AI models designed to generate new data samples that resemble the training data. These models can create new text, images, music, or other content.
Neural Networks
A set of algorithms modelled loosely after the human brain, designed to recognize patterns.
Tags
- Additive Manufacturing